Posted By: Alyson Barnes, PhD, Assistant Editor, AJHG
Each month, the editors of The American Journal of Human Genetics interview an author of a recently published paper. This month we check in with Kendall to discuss her recent paper “Evaluating large language models on medical, lay language, and self-reported descriptions of genetic conditions.”
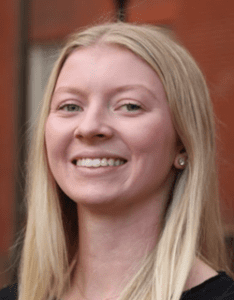
AB: What motivated you to start working on this project?
KF: Our research group’s primary focus is to use artificial intelligence and deep learning-based approaches to study clinically-relevant genetics questions. This specific project is motivated by the rapid increase in the use of large language models (LLMs) for a variety of purposes, including research, education, writing, content creation, and in medical settings. LLMs are incredibly impressive at accomplishing a wide variety of natural language processing tasks and they have great potential to be useful tools in medical spaces due to their ability to analyze and respond to questions in a user-friendly interface. However, these LLMs are still relatively underexplored in the field of medical genetics and genomics. To begin exploring how these LLMs perform in this area, we completed a preliminary study in 2023 comparing ChatGPT to humans on a variety of genetics questions. The 2023 paper stirred some interesting discussion and opened the door to further questions about LLMs in this context. Therefore, our primary motivation for this recent paper was to assess the capabilities of not just the best performing and most well-known LLMs, but also some of the smaller, open-source LLMs, as well as a domain-specific medical LLM, to assess the strengths and weaknesses of these different models and their diagnostic abilities in medical genetics. We also wanted to explore how LLMs could be used by individuals who are not necessarily medical or scientific professionals. Finally, we also assessed how LLMs could respond to real patient queries. We are excited to continue exploring the intersection of LLMs and medical genetics and trying to understand how these tools can be improved and best used in medical settings.
AB: What about the papers/project most excites you?
KF: I am excited about so many parts of this project! This study is a preliminary exploration of the role of LLMs in medical genetics, so I am most excited about expanding on our findings and exploring the new ways that LLMs and other AI-based tools can be assessed and improved in medical settings. In terms of our results, I am most excited by the findings that LLMs are able to respond accurately to medical genetics questions phrased in both medical and layperson language. The fact that LLMs can respond accurately to questions that don’t use specific medical language is exciting because it provides the opportunity for LLMs to be used by individuals without extensive or formal medical knowledge. Other tools like Google searching appear to rely more directly on specific medical terminology to retrieve the most accurate medical information, and users have to parse through thousands of Google results to get their questions answered accurately. Although they still must be improved, LLMs may eventually provide a way to expand access of medical knowledge and diagnostic abilities to individuals who don’t have a medical background and to medically underserved areas, and this is an exciting prospect.
AB: Thinking about the bigger picture, what implications do you see from this work for the larger human genetics community?
KF: LLMs and other deep learning-based tools are already being used in clinical settings, so it is important to understand how they might respond in various contexts, what their strengths, weaknesses, and biases are, and how they can be improved. Our work is meant to be a preliminary benchmark of how these tools perform in the context of human genetics so that other researchers, clinicians, and patients can be aware of their abilities in clinical settings. For example, our work has shown that LLMs are very competent at providing differential diagnoses and responding to textbook-style standardized questions that represent the most common phenotypes of genetic conditions. However, when real patients were asked to describe the manifestations of their conditions, LLMs have a much more difficult time providing accurate differential diagnoses and information, as real patient presentations do not always follow the most common phenotypes or “textbook descriptions”, and individuals may describe themselves in various ways. This indicates that while LLMs are impressive in medical settings, they may be less ready to be used directly with patient-written questions without oversight. We hope that this study will show the human genetics community that for these models to be clinically useful, we need to increase the amount of data and the data must reflect the diversity of patients across the globe. This includes not only increased data on all medical conditions, but also variation in areas like race, ethnicity, gender, and age, as well as many other factors to increase the usefulness of these tools for all people. LLMs have the impressive ability to be able to analyze language in a clinically useful way and expand access to accurate medical genetics information to individuals without medical knowledge or access to genetics care, so we hope that our study will help increase understanding about where these tools are currently, how we can improve them, and their potentially transformational abilities in genetics. We already know that AI is being used in clinical settings. Now, the key questions are determining where and how AI should be implemented in patient care, as well as identifying areas where it should be avoided to ensure the highest quality of care.
AB: What advice do you have for trainees/young scientists?
KF: As a trainee/young scientist myself, I still have lots to learn! However, one of the biggest lessons I have learned in my early career is that failures are just as valuable as the successes. That is, if an experiment or an exciting idea does not work as you expect, there can be just as many valuable lessons and insights that can come of that result as compared to a ‘successful’ result. Because of this, I have learned to not be afraid to try new ideas and explore new angles of approaching a research question. This mindset has greatly expanded my passion for research. I am extremely grateful to my entire research group and my mentors Dr. Ben Solomon and Dr. Dat Duong for helping me reframe the notion of success and failure in research and for being so supportive and helpful while also allowing me to investigate my own research ideas.
AB: And for fun, tell us something about your life outside of the lab.
KF: I’m from Florida and growing up there made me a huge nature and animal lover! I love spending my free time outdoors, whether I’m hiking, walking, or running, and I enjoy learning about different animal species.
Kendall Flaharty, BS is a Postbaccalaureate Fellow at the National Human Genome Research Institute (NHGRI) at the National Institutes of Health (NIH).