Posted By: Kylee Spencer, PhD, Assistant Editor, AJHG
Each month, the editors of The American Journal of Human Genetics interview an author of a recently published paper. This month, we check in with Yun Li and Quan Sun to discuss their recent paper, “MagicalRsq-X: A cross-cohort transferable genotype imputation quality metric.”
KS: What motivated you to start working on this project?
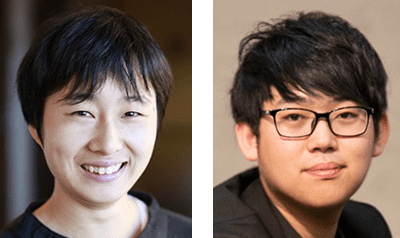
YL and QS: Genotype imputation is a standard practice but not all variants can be well imputed. State-of-the-art approaches perform poorly for low frequency variants (LFVs) and rare variants (RVs), either removing well-imputed variants or failing to filter out poorly imputed variants. With increasingly large reference panels, these LFVs and RVs constitute >90% of imputed variants and thus pose a pressing challenge. To address this challenge, we previously published MagicalRsq. MagicalRsq proves to aid post-imputation quality control for LFVs and RVs. However, it requires additional genotype data in the target cohort other than those used when performing imputation. Although many studies have subsets of their samples sequenced or genotyped with a separate genotyping platform, the vast majority of studies still have only one set of genotype array data for their samples. This major limitation of MagicalRsq motivated us to develop MagicalRsq-X, which circumvents the issue by harnessing the power of many cohorts with whole genome sequencing (WGS) data.
KS: What about this paper/project most excites you?
YL and QS: We are most excited to observe that our MagicalRsq-X models exhibit broad transferability across diverse cohorts, as shown in multiple TOPMed cohorts and the Cystic Fibrosis Genome Project, for both individuals with European ancestry and African Americans. These observations suggest that our pre-trained models will broadly benefit many studies without any extra genotype data beyond those used when performing imputation.
KS: Thinking about the bigger picture, what implications do you see from this work for the larger human genetics community?
YL: I strongly advocate using MagicalRsq-X for post-imputation quality control. We released our pre-trained models as well as all other input features except the standard Rsq, to make it maximally straightforward for investigators to apply the models to their own data. I really hope that this simple MagicalRsq-X add-on step will become standard practice. As demonstrated in the paper, I believe that this simple change will rescue many LFVs and RVs associated with various complex traits missed without MagicalRsq-X-recalibrated imputation quality scores, expediting the discovery of causal variants in the lower frequency domain, thus potentially revealing novel drug targets and advancing personalized medicine.
QS: I would like to add that MagicalRsq-X involves borrowing information across cohorts, which also brings up a question of what reference to use as a training cohort. This is a very common problem that we have seen in many different analyses, including ancestry inference, polygenic risk predictions, etc. In this work, we provided a simple ad hoc solution of using harmonized genotype principal components (PCs), which is of course far from perfect. I hope the broader human genetics community could think more about such problems when using “references”, and I would also like to emphasize the use of accurate population descriptors to minimize loss due to reference mismatch.
KS: What advice do you have for trainees/young scientists?
YL: I would like to encourage trainees/young scientists to focus more on solving important problems than chasing after buzzwords. The XGBoost model was proposed ~8 years ago and genotype imputation was first proposed almost two decades ago. Coupling the two together, MagicalRsq-X provides a valuable tool that can discover biologically and clinically meaningful variants for complex diseases and traits.
QS: As a trainee and young scientist myself, based on my own experience, I would say that hands-on experience working with real datasets is a great way to identify biological problems. This is the case for our original MagicalRsq project, where we identified the issues of imputation quality estimates for the Cystic Fibrosis Genome Project. Exploring more deeply, we further extended our MagicalRsq to this MagicalRsq-X for broader applicability.
KS: And for fun, tell us something about your life outside of the lab.
YL: I always enjoy biking and origami, the former helping me stay active and the latter helping me to either rest my brain by switching to an idle state or to think without Internet or screen “contamination”. My latest hobby is to explore (well, largely watching the rest of my family generate) AI-aided artwork.
QS: I am a classical pianist beyond my scientific career. I started playing the piano when I was 4 years old and really appreciate what music provides. It not only helps my brain to relax but also leads to a spiritual world to express myself and experience all different kinds of feelings that I cannot describe in words. Music supported me through many hardships, especially during COVID-19 lockdown. By the way, I will be giving a piano recital at UNC-Chapel Hill in May!