Written by Nascent Transcript writer Sumantra Chatterjee, PhD
February 2018
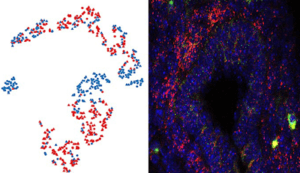
Left panel: A tSNE plot showing cell trajectories and fates from E12.5 mouse gut cells sorted on expression of the gene Ret.
Right panel: An immunofluorescence image of a transverse section of E12.5 mouse gut labelled for Ret expressing cells (red).
Almost 180 years ago, Theodor Schwann, Matthias Schleiden, and Rudolf Virchow laid down three fundamental tenets of biology collectively called the “cell theory”. Since then, the principles that the cell is the most basic unit of life, all living organisms are composed of cells, and all cells arise from preexisting cells have been the bedrock of biology. As we have continued to study and understand the basic properties of living organisms, both in normal and disease conditions, we have interrogated these systems starting at the whole organismal level and working our way down to tissue and groups of cells.
The findings from these studies have expanded our understanding of how biological systems function, but have stopped short of comprehensively answering some fundamental questions—How many different types of cells are there in a given organism? How does this diversity arise? And how do these diverse cells interact in a tissue and influence how tissue impacts the organism? Recent advances in our ability to both isolate and experimentally manipulate single cells have led us to answer some of these questions in a more systematic and quantitative manner than previously possible.
The challenges we face in classifying the various cell types of an organism are daunting when considering cells’ developmental trajectories. Each cell in an adult organism arises from a zygote (a fertilized egg) through a sequence of cell divisions and fate transitions driven by intricate gene expression changes, which result from a multitude of morphogen gradients and signaling. Capturing this dynamic process for each individual cell from datasets derived from bulk tissues has been a challenge.
Expression levels of genes is just one of the many biological phenomena that allow us to determine a cell’s state. Among different cell types, different chromatin regions are accessible for regulation and transcription (measured by ATAC-seq and DNase-seq), methylation marks differ (measured by whole genome bisulphite-seq), and different regions of the genome are in contact (measured by HiC and ChIA PET). Initial expression studies measured these processes in bulk cells and tissues, which conveyed an average picture that was not helpful in delineating the range of interactions occurring at the individual cell level.
In the last five years, we have made remarkable progress in scaling down most of these experimental protocols to single cells. The ability to assay the expression level of every gene across thousands of individual cells has given us an unprecedented look at how diverse these processes are, even in cells that were assumed to be identical, and allowed us to capture minute changes as a cell transitions from one state to the next. These experiments can be performed on all cells in a given tissue without the need to experimentally purify them, and thus, eliminates the need for cell type specific marker genes. Further, single cell expression measurements can distinguish different cell types, as opposed to bulk measurements, which generally reflect average values across cell types.
The two biggest challenges of assaying single cells are 1) the ability to sequence with a limited amount of biological material and 2) the analysis of post-sequencing data, which can be extremely sparse. With regards to the former, major technical advances over the last couple of years have made generating sequencing libraries from picograms of biological materials more robust and reproducible. As more scientific groups with varied expertise enter the realm of single cell genomics, these processes will hopefully be further refined and become standardized.
As for the latter challenge, analyzing single cell RNA-seq data is substantially more difficult than bulk experiments. The size and scale of the data puts considerable strain on most analysis pipelines, and single cell expression measurements are often highly variable and sparse. Thus, distinguishing true biological variation from technical noise is not a trivial task. New normalization techniques, or “barcoding” of cells, are needed to attribute changes in expression across diverse cell populations. Also, as more such experiments are conducted, and at scales larger than ever before, new algorithms and statistical tests will be necessary to detect minute biological variations in a sea of large technical noise.
Single cell genomics is revolutionizing our understanding of genomic processes by making data available at a scale previously unimaginable. Robust clustering analysis will help catalog cell types, states, and transitions. In time, we may be able to construct complete dynamic gene regulatory networks as cells undergo transitions and fate changes. We are at the beginning of an exciting journey during one of the most innovative times in biology where we are able to answer questions previously thought impossible. However, much work remains to be done, both in the experimental and computational domains as we come across challenges we have not previously encountered. There are still aspects of genome regulation, such as transcription-factor binding activity, that are not yet measurable in single cells. Additionally, many basic statistical questions, such as batch effects and technical variability, need to be properly addressed.
As both the scale and scope of our questions increase, we will be forced to think outside the box to come up with new techniques and solutions. As the genetics field attracts people with diverse skills, such as biologists, chemists, engineers, and statisticians, we will need to pool our resources and expertise to untangle the many knotty problems posed by our genome and to finally completely understand the very basic unit of our life—The Cell.